How to Verify On-Chain Machine Learning Algorithms Using Zero-Knowledge Proofs?
Mar 25, 2025
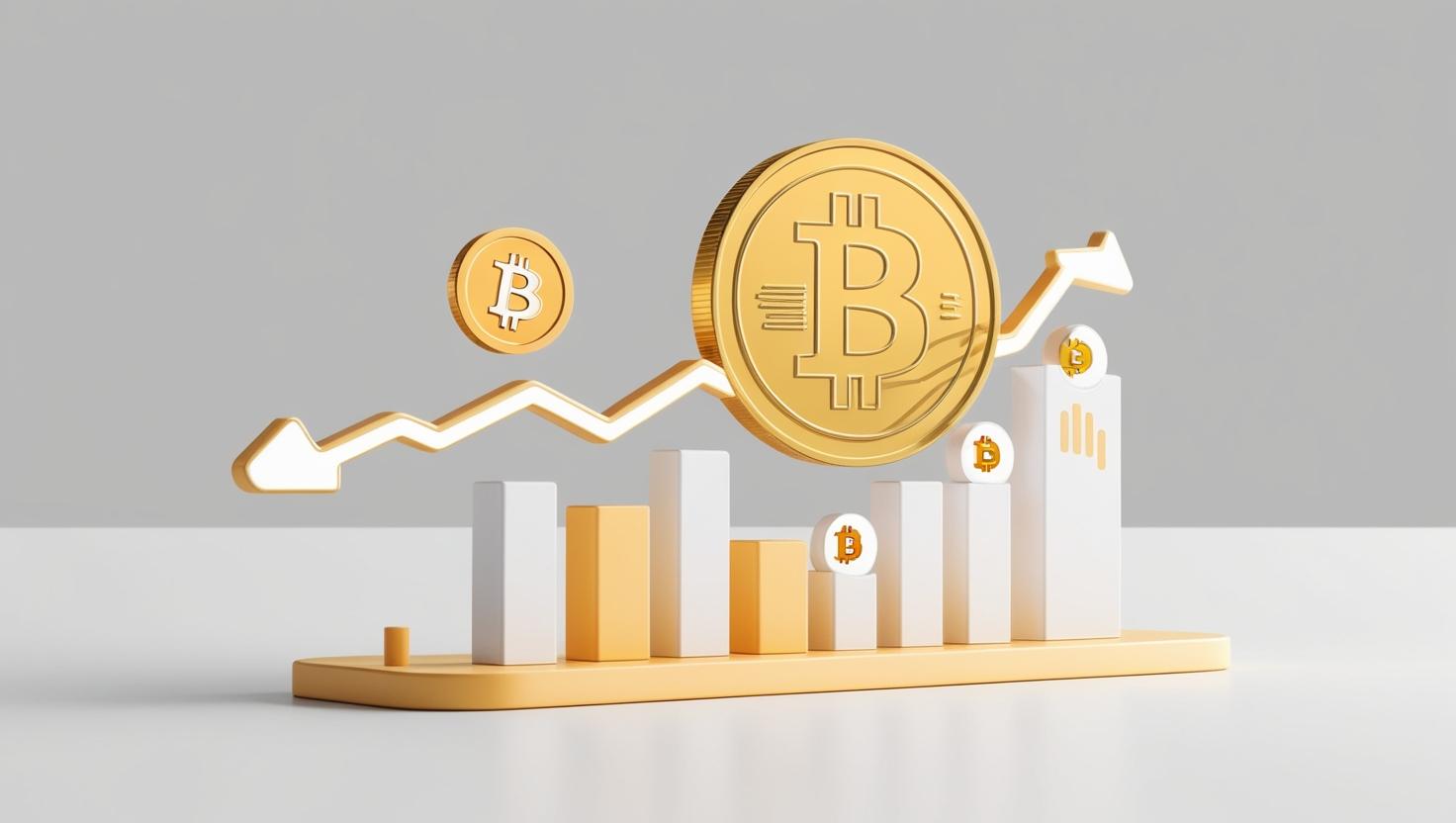
The integration of machine learning (ML) with blockchain technology presents exciting opportunities, particularly in enhancing data privacy and security. However, the verification of on-chain ML algorithms poses significant challenges, primarily due to the need to ensure data integrity and model validity without compromising sensitive information. Zero-knowledge proofs (ZKPs) emerge as a powerful solution, allowing one party to prove knowledge of a fact without revealing the information itself. This blog post explores how ZKPs can be utilized to verify the correctness of ML algorithms deployed on-chain. By leveraging this cryptographic technique, developers can enhance trust in ML models while maintaining the confidentiality of the underlying data.
Understanding Zero-Knowledge Proofs
Zero-knowledge proofs are a revolutionary concept in cryptography that allows for the verification of information without disclosing the actual data. This ensures that sensitive data, such as training datasets or model parameters, remains private even while proving correctness. The fundamental idea is that one party, known as the prover, can demonstrate knowledge of a specific piece of information to another party, the verifier, without sharing that information. This is particularly useful in scenarios where data privacy is paramount, such as in healthcare or financial services. By applying ZKPs to on-chain machine learning, it becomes possible to validate algorithms while safeguarding confidential data.
ZKPs provide a mechanism for verifying computations without revealing inputs.
They allow for the secure sharing of model insights without exposing the model itself.
The technology can help in building trust among stakeholders in a decentralized environment.
ZKPs can significantly reduce the risk of data leakage during the verification process.
This cryptographic approach enhances the security of smart contracts used in ML applications.
The Role of Machine Learning in Blockchain
Machine learning has the potential to revolutionize numerous sectors by providing predictive analytics and enabling data-driven decision-making. When integrated with blockchain, ML can enhance processes such as fraud detection, risk assessment, and personalized services. However, the deployment of ML algorithms on decentralized platforms introduces unique challenges, particularly regarding transparency, accountability, and data privacy. Ensuring that these algorithms are functioning as intended without revealing sensitive data is crucial for gaining user trust. As a result, the intersection of ML and blockchain necessitates robust verification mechanisms, making ZKPs an attractive solution.
ML can identify patterns in large datasets that traditional methods may overlook.
Combining ML with blockchain ensures that the data used is immutable and tamper-proof.
The decentralized nature of blockchain enhances the transparency of ML algorithms.
Users can benefit from personalized services while preserving their privacy.
The integration of these technologies fosters innovation across various industries.
Implementing Zero-Knowledge Proofs in ML Verification
To implement ZKPs for verifying on-chain ML algorithms, several steps must be taken. First, the ML model must be designed in a way that allows for the generation of proof statements. These statements should articulate what the model is supposed to accomplish without revealing the underlying data or logic. Next, the prover will generate a zero-knowledge proof that the algorithm has been executed correctly based on the input data. This proof is then sent to the verifier, who can validate the accuracy without needing to access the original data. This process not only secures the verification but also aligns with the principles of decentralized trust.
Define the outputs of the ML model clearly to facilitate proof generation.
Use cryptographic algorithms that support the creation of zero-knowledge proofs.
Ensure that the proof is computationally efficient to avoid delays in verification.
Establish a robust protocol for transferring proofs between the prover and verifier.
Continuously test and validate the implementation to address potential vulnerabilities.
Challenges and Considerations
While the application of ZKPs in verifying ML algorithms offers numerous advantages, there are also challenges that need to be addressed. One significant hurdle is the computational overhead associated with generating and verifying proofs, which can be resource-intensive. Additionally, ensuring that the ML models are robust against adversarial attacks while still being verifiable presents another layer of complexity. Developers must also consider the scalability of ZKP implementations, particularly as the size of the data and models increases. Finally, regulatory compliance and ethical considerations regarding data privacy must be upheld throughout the process.
Proof generation can be resource-heavy, impacting overall system performance.
The design of ML models must incorporate security against potential threats.
Scalability remains a concern as data volumes grow in the blockchain environment.
Developers must navigate the evolving landscape of data privacy regulations.
Ethical considerations should guide the deployment of ML on blockchain platforms.
The Future of On-Chain Machine Learning with ZKPs
The future of on-chain machine learning with zero-knowledge proofs looks promising as the demand for secure, privacy-preserving solutions continues to rise. Innovations in ZKP technology are expected to reduce the computational burden and improve efficiency, making it easier to implement these proofs in real-time applications. Furthermore, as more industries recognize the value of combining AI and blockchain, the need for robust verification mechanisms will grow. This convergence will likely lead to new use cases and applications that can enhance trust and transparency in various sectors. Ultimately, the synergy between ML and ZKPs may pave the way for a more secure and privacy-conscious digital landscape.
Ongoing advancements in ZKP technology will enhance usability and performance.
Industries will increasingly demand secure solutions for data handling and model verification.
New applications will emerge, driven by the combination of ML and blockchain.
Trust and transparency will become central tenets of future digital ecosystems.
The collaboration of developers, researchers, and regulators will be essential for success.
Start your SAFE cryptocurrency journey now
Fast and secure deposits and withdrawals, OSL safeguards every transaction !